Details of Tesla’s FSD V12 Development Revealed: Over 10 Million Video Inputs in 8 Months, Sometimes Outperforming Musk
5 min read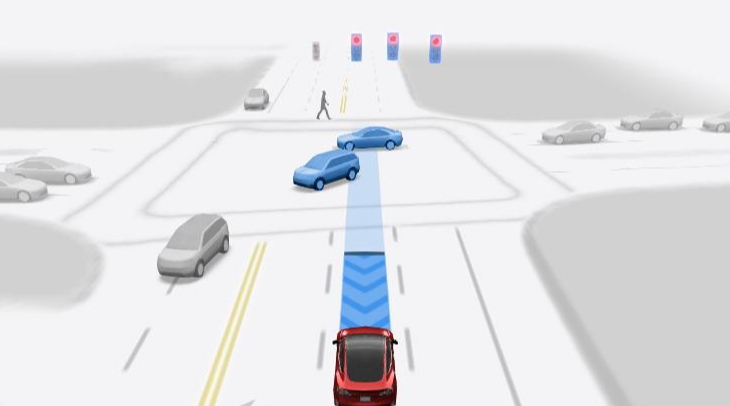
Every day, 160 billion video frames are used for training
The development details of Tesla’s FSD V12 system have been unveiled.
While Elon Musk had previously hinted at a change in the FSD V12 technology roadmap, it is surprising to learn that Tesla only began training this neural network-based intelligent driving algorithm in early this year.
Just four months later, the new system was ready to replace the old one, and after eight months, the brand-new FSD V12 made its debut during Musk’s live presentation.
Behind this lies a change in the technology roadmap, moving from rule-based to data-driven, and from modular design to end-to-end.
It has also brought new challenges.
FSD V12: All About Neural Networks
In essence, Tesla’s FSD V12 has a single core feature: there is no rule-based code, only neural networks.
What does this mean?
Most common autonomous driving systems on the market employ a modular design, consisting of three main modules: perception, decision-making, and control. Each task within these modules uses its own algorithmic models.
AI algorithms are primarily applied in the perception module, while the decision-making and control modules remain conventional, based on if-else logic code.
In other words, code written by algorithm engineers establishes a set of rules for the autonomous driving system. For example, it dictates that the car must stop at a red light, proceed at a green light, and maintain a lane position, among other things.
The drawback of such a system is evident: the rule-setting standards are determined by various engineers, and the driving style can easily mismatch a driver’s preferences, resulting in a poor user experience that is often worse than manual driving.
With Tesla’s FSD V12 being all about neural networks, it means that the usual perception, decision-making, and control modules are no longer required in the design. Instead, the focus is on defining the neural network architecture and then training it with input data.
A single neural network can process all input signals and output driving decisions.
Based on real human driving data, the system learns how to drive and continually improves. This transition represents the shift from rule-based to data-driven.
In the past, systems used rules to determine how to drive based on various environmental inputs. Now, during training, human driving data is input, and the system thoroughly learns human driving habits. In real-world driving situations, it autonomously decides how to drive based on environmental inputs.
If there are situations where it doesn’t perform well, additional data is input specifically for those scenarios.
This training approach is similar to that used for models like ChatGPT but adapted for automotive applications.
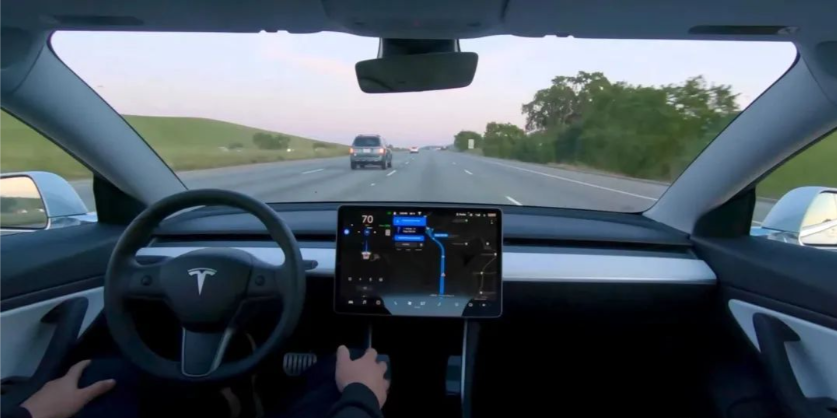
Before deciding to change its technological roadmap, Tesla’s autonomous driving team demonstrated to Elon Musk that the neural network-based system could handle certain situations better.
When there were trash cans, fallen traffic cones, and random obstacles on the road, the car accurately maneuvered around these obstacles, crossed lane lines, and occasionally violated some traffic rules when necessary.
Prior to the live presentation, Musk also tested the FSD developed based on neural networks.
During a 25-minute drive, Musk only pressed the accelerator when the system was overly cautious but never touched the steering wheel. Additionally, there was one instance where the system performed better than he had expected.
“My human neural network failed here.”
How to Interpret
In fact, the concept of end-to-end autonomous driving systems had already gained traction among players in the autonomous driving industry before Musk announced the transition to FSD V12’s end-to-end technology roadmap.
End-to-end autonomous driving system development is less challenging as it doesn’t require writing massive amounts of code upfront (FSD V11’s control stack had over 300,000 lines of C++ code) or engineers to design rules in advance.
Instead, all that’s needed is to continuously input human driving data, and the system learns autonomously by observing.
However, this approach imposes high demands on autonomous driving players as well.
For example, the input data must be of high quality to better assist the system in learning. Musk found that the neural network-based autonomous driving system started performing well only after inputting over a million videos.
At the beginning of this year, Tesla had already fed this system with 10 million human driving videos, which were carefully selected to represent experienced drivers.
Tesla’s global fleet of nearly 2 million vehicles also provides approximately 160 billion video frames for training every day. Tesla expects that the volume of video used for training will reach billions of frames in the future.
This presents challenges related to data volume, data labeling, computing power, and more.
Furthermore, the key challenge with end-to-end technology is its inherent lack of explainability. Currently, end-to-end autonomous driving remains a “black box” with no precise way to explain why the system performs poorly in specific situations.
Tesla’s proposed solution is to feed the system more data when it encounters situations where it doesn’t perform well. For example, when the system almost ran a red light during Musk’s live presentation, the solution was to input more videos of traffic signals, especially left turn signals.
Additionally, Musk set a metric for the team: to display in real-time the number of miles driven by the FSD system without human intervention. If interventions occur, the corresponding issues are addressed.
More importantly, this ongoing learning process introduces a new challenge: the system learns not only smooth operations from experienced drivers but also instances where human drivers deviate from traffic rules.
For example, when encountering a stop sign, over 95% of people slow down and proceed rather than coming to a complete stop.
This means that regulatory bodies will need to establish clear standards.
The National Highway Traffic Safety Administration in the United States is currently researching whether to permit autonomous driving systems to perform operations that do not fully comply with traffic regulations.
In summary, the introduction of Tesla’s FSD V12 is indeed significant for autonomous driving. Since it can achieve full AI integration throughout the process, it opens up possibilities for moving toward AGI, or general artificial intelligence.
The moment when autonomous driving reaches the equivalent of ChatGPT may be approaching, and the gears of fate may be starting to turn.